The Transformative Power of Labelling Tool Machine Learning for Business
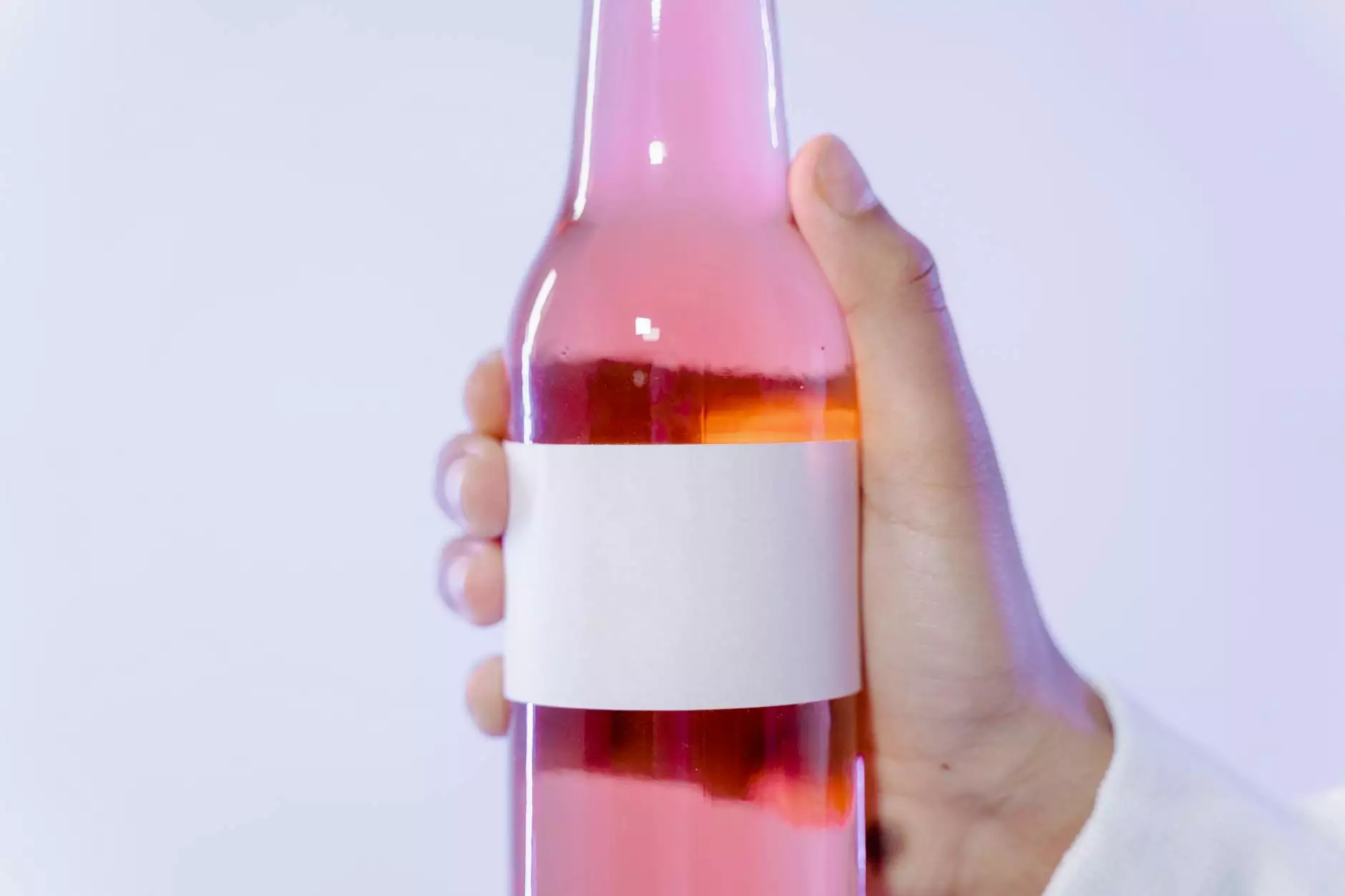
In today's fast-paced digital landscape, businesses are constantly seeking ways to enhance efficiency, streamline operations, and leverage data for informed decision-making. One of the most revolutionary advancements in recent years has been the integration of machine learning into various sectors, including Home Services, Keys & Locksmiths. A critical component in this domain is the labelling tool machine learning, which plays a pivotal role in ensuring that algorithms learn effectively and produce reliable results.
Understanding Machine Learning and Its Importance
Machine learning is a subset of artificial intelligence (AI) that empowers systems to learn from data, identify patterns, and make decisions without explicit programming. It has transformed industries by providing insights that were previously unreachable through traditional data analysis methods. For businesses, this means improved customer service, optimized logistics, and enhanced product development.
What are Labelling Tools?
Labelling tools are software applications or frameworks that assist in the process of tagging or annotating data. In the context of machine learning, particularly in supervised learning, data needs to be labelled to train algorithms effectively. Accurate labelling ensures that the machine learning models can understand the data context and make informed predictions based on it.
Key Functions of Labelling Tools
- Data Annotation: Labelling tools facilitate the manual or automated annotation of data, which is crucial for training models.
- Quality Assurance: Many labelling tools come with features to verify the accuracy of labels, ensuring data quality.
- Collaboration: These tools often enable teams to collaborate efficiently, allowing multiple users to work on labelling tasks concurrently.
- Integration: Labelling tools can often integrate with machine learning frameworks, simplifying the workflow.
The Relevance of Labelling Tool Machine Learning in Business Operations
Businesses must adapt to the dynamic market conditions while keeping an eye on efficiency and cost-effectiveness. The labelling tool machine learning provides the foundation for organizations to harness the power of data effectively, offering several benefits that drive operational excellence.
1. Enhanced Data Handling
With an influx of data generated by various business operations, the ability to catalogue and label information becomes paramount. Labelling tools streamline the data handling process, allowing businesses to focus on critical decision-making based on well-structured datasets. For example, in the Keys & Locksmiths industry, accurately labelled data about customer preferences and job histories can lead to improved service design and customer satisfaction.
2. Improved Model Performance
For machine learning models to operate efficiently, they require high-quality, well-annotated data. Labelling tools ensure that the training datasets are comprehensive and accurate, which in turn facilitates better performance of the algorithms. Businesses leveraging machine learning in their Home Services operations can see significant improvements in models predicting maintenance needs or customer behavior.
3. Streamlined Workflows
By integrating labelling tools into machine learning workflows, businesses can achieve greater efficiency. Automated labelling methods reduce the time spent on manual annotations, helping teams to focus on strategy and execution. This results in faster deployment of AI models, enhancing operational agility.
4. Cost Efficiency
Investing in effective labelling tools also translates to cost efficiency. By improving the speed and accuracy of data processing, businesses can reduce the manpower required for data preparation and thereby lower operational costs. Fewer resources spent on labelling means more funds can be allocated toward other critical business areas.
Challenges in Data Labelling
Despite the numerous benefits of labelling tools, businesses may encounter challenges. Understanding these challenges allows for better strategy formulation and implementation.
1. The Complexity of Labelling
Some datasets may require complex labelling strategies, especially in industries where customer interactions generate diverse forms of data. Familiarity with the nature of data is essential to devise an effective labelling format. For instance, in Home Services, data about service history, customer feedback, and inventory must be labelled in a way that captures the variances and intricacies of each service request.
2. Resource Allocation
Effective data labelling can be resource-intensive, as it often demands skilled personnel to ensure quality. Businesses must strike a balance between using in-house resources and outsourcing data labelling tasks to maintain productivity without compromising on quality.
3. Technology Integration
The integration of labelling tools with existing machine learning frameworks can sometimes be challenging. Ensuring compatibility and functionality requires careful planning and execution. It's imperative for organizations to choose labelling tools that can seamlessly integrate with their machine learning systems to mitigate these issues.
Best Practices for Implementing Labelling Tools
To maximize the potential benefits of labelling tool machine learning, businesses should adhere to established best practices that ensure effective implementation.
1. Define Clear Labelling Guidelines
Establishing clear criteria for labelling is fundamental. Teams should outline the necessary information required for each data point and develop a consistent format to eliminate ambiguity, enabling consistency across the board.
2. Utilize Automated Labelling Where Possible
While manual labelling is sometimes unavoidable, leveraging automated labelling technologies can save time and reduce human error in data annotation. Using algorithms to pre-label data can accelerate projects significantly, with teams conducting reviews and corrections only when necessary.
3. Conduct Regular Training
Investing in training programs for personnel involved in data labelling ensures that they are well-versed with the tools and understand the importance of accurate labelling. Continuous education keeps the team updated on the latest best practices in machine learning and data management.
4. Monitor and Evaluate
Regular assessment of the labelling process and its outcomes is crucial. Employing key performance indicators (KPIs) to monitor the quality and impact of labelled data helps identify areas for improvement and ensures the labelling efforts align with business goals.
Future Trends in Labelling Tool Machine Learning
The future of labelling tools in machine learning holds exciting prospects. As technology continues to evolve, several trends are expected to shape the landscape.
1. Increased Use of AI in Labelling
Advancements in AI are leading to more sophisticated labelling tools that can learn from past labelling patterns, improving their efficiency and accuracy. These AI-driven tools will become integral in dealing with large datasets commonly found in sectors like Home Services and Keys & Locksmiths.
2. Greater Emphasis on Collaboration
Collaborative labelling platforms are on the rise, allowing teams to work together efficiently from different locations. Features that facilitate real-time collaboration and feedback will enhance productivity and improve data quality.
3. The Rise of Cloud-Based Solutions
As businesses migrate to cloud storage and computing, labelling tools will increasingly adopt cloud-based solutions. This trend will provide greater flexibility and accessibility, allowing teams to manage and label data from anywhere at any time.
4. Ethical Considerations in Data Labelling
As awareness of data ethics grows, businesses will need to consider how to ensure ethical labelling practices. This includes data privacy, consent, and the potential biases that may arise during the labelling process. Transparency and responsibility in data handling will become paramount.
Conclusion: Empower Your Business with Labelling Tools for Machine Learning
The integration of labelling tool machine learning is more than just a trend; it’s a necessity for businesses eager to enhance their operational capabilities and stay ahead in a competitive market. By embracing these tools, companies in sectors like Home Services and Keys & Locksmiths can create robust machine learning models that drive informed decision-making and elevate customer experiences. As businesses navigate through complexities, it becomes clear that effective data labelling is the backbone of successful machine learning initiatives. Investing in these technologies will position businesses for a future where data-driven strategies become the norm, fundamentally reshaping how operations are conducted.